Building deep neural networks to detect candy from photos and estimate nutrient portfolio
(1) John Burroughs School, (2) Brown School, Washington University in St. Louis
https://doi.org/10.59720/22-242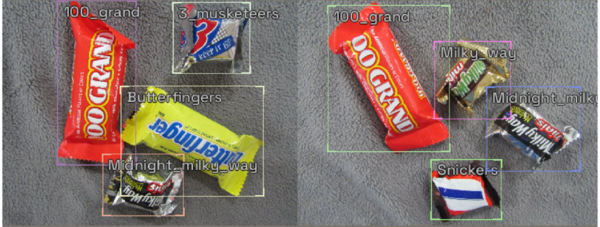
Approximately one third of American youth consume candy on a given day. Consuming excess candy contributes to added sugar intake and may lead to tooth decay and other health concerns. Diet-tracking apps may inform and help regulate candy consumption but depend on the availability of annotated candy image data and predictive models. A recent review documented differences in daily intakes of calories and macronutrients between app predictions and ground truths ranging from 1.4%–10.4%. Transfer learning-based deep neural networks could outperform those benchmarks and reduce the error margin. We built a dataset of 1,008 images comprising nine common candy types and developed four neural network models to detect candy pieces. The best-performing model achieved a mean average precision of 0.8736 for localizing candy pieces of different types in the validation dataset and an accuracy of 99.8% for predicting the quantity and types of multiple candy pieces in the test dataset. By combining candy type-specific nutritional information obtained from the nutrition facts label, the model accurately estimated (within an error margin of 0.5%) the aggregate nutrient portfolios, including total calories, total fat, saturated fat, cholesterol, sodium, carbohydrates, total sugars, and added sugars, of all candy pieces shown in an image. This study demonstrates the feasibility of automating candy calorie/nutrition counting using photos, which may facilitate the development of diet-tracking apps to provide real-time, accurate nutritional information to inform candy consumption.
This article has been tagged with: