Entropy-based subset selection principal component analysis for diabetes risk factor identification
(1) Sekolah Menengah Atas (SMA) Santo Paulus Pontianak, (2) Informatics, Universitas Widya Dharma Pontianak
https://doi.org/10.59720/23-015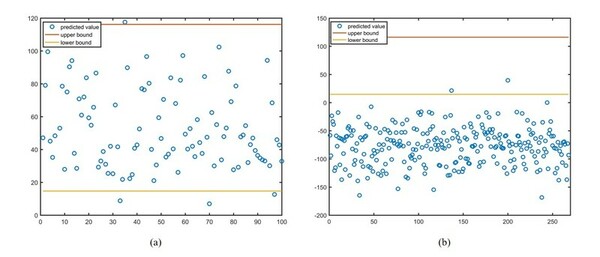
Diabetes is one of the most common diseases, with an estimated 10% of the population suffering from this disease. Therefore, it is important to be able to identify the symptoms of this disease as early as possible before the patient's condition worsens and requires more expensive medical treatment. We aimed to study whether the entropy-based subset selection principal component analysis (E-ss PCA) can diagnose if a person is diabetic. The E-ss PCA is a novel machine learning method that can identify important parameters from a dataset. The E-ss PCA method was originally developed to fix the linearity problem that occurred when the principal component could not be written as the linear combination of the original parameters. Through the process, the E-ss PCA generates subsets of data that guarantee the linearity of variables of the subset. Via the E-ss PCA algorithm, we aim to verify which diabetic risk factors, such as pregnancy, triceps skinfold thickness, Body Mass Index (BMI), pedigree function, and age, are significant. Based on a dataset of diabetes patients from the United States National Institute of Diabetes and Digestive and Kidney Diseases, the E-ss PCA method was able to predict whether a person has diabetes or not with an average accuracy of 97.30%, which is higher than the classical PCA with an average accuracy of 94.45%. Furthermore, the proposed algorithm identified that the risk factors that accurately predict diabetes are BMI, triceps skin fold thickness, and blood pressure.
This article has been tagged with: