Automated classification of nebulae using deep learning & machine learning for enhanced discovery
(1) Mission San Jose High School, (2) Senior Mentor
https://doi.org/10.59720/23-105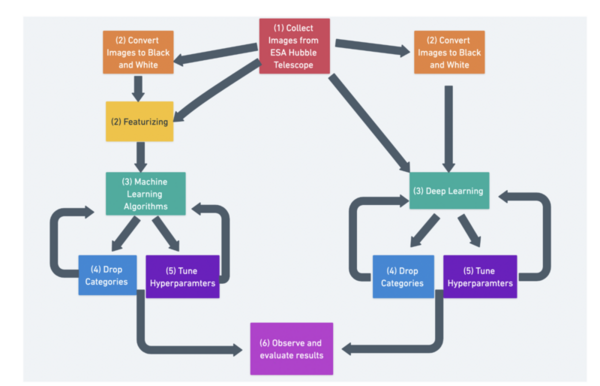
There are believed to be ~20,000 nebulae in the Milky Way Galaxy. However, humans have only cataloged ~1,800 of them even though we have gathered 1.3 million nebula images. Resources like the Hubble telescope can automatically explore space, discover new artifacts, and capture new images. Still, their classification is a human skill, which ultimately is interminable and subject to human error. Classification is important as it helps scientists understand the chemical composition of a nebula which in turn helps them understand the material of the original star. Our research on nebulae classification aims to make the process of classifying new nebulae faster and more accurate using a hybrid of deep learning and machine learning techniques. Our hypothesis is that the application of machine learning and deep learning methodologies effectively classifies nebulae based on images. Using a dataset primarily of images from the European Space Agency, we experimented with a range of artificial intelligence techniques such as featurization and color conversion and then determined the deep learning network/machine learning algorithms that produced the best results. The main conclusions reached from our research were that (a) the artificial intelligence (AI) was not dependent on color to classify nebulae, (b) dropping specific categories increased accuracy, and (c) featurization is the most effective technique to classify nebulae accurately. Automated classification of nebulae will help us discover, identify, and classify these marvels much faster and more accurately to expand our understanding of nebulae.
This article has been tagged with: