Analyzing breath sounds by using deep learning in diagnosing bronchial blockages with artificial lung
(1) Bellarmine College Preparatory, (2) Rambus Inc.
https://doi.org/10.59720/22-004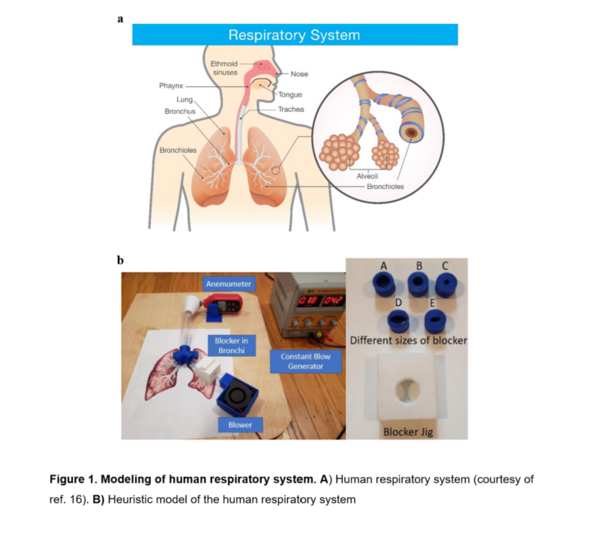
Many common respiratory illnesses like bronchitis, asthma, and chronic obstructive pulmonary disease (COPD) lead to bronchial inflammation and, subsequently, a blockage. However, there are many difficulties in measuring the severity of the blockage. To detect the blockages associated with these illnesses, most medical staff have relied on patients’ descriptions of their symptoms or the doctor’s experience or monitoring through a medical device like the stethoscope. However, these diagnostic measures are error-prone and time-consuming, leading to frequent misdiagnosis and limitations in continuous perioperative monitoring. Therefore, a numeric metric to determine the degree of the blockage severity is necessary. To tackle this demand, we aimed to develop a novel human respiratory model and design a deep-learning program that can constantly monitor and report bronchial blockage by recording breath sounds in a non-intrusive way. A bronchial lung model would provide doctors or medical staff with a better understanding of patients’ conditions and allow faster more targeted treatment. Performing these experiments demands a new design of the artificial respiratory system. Alternative to using human testing, there is more accuracy in characterizing human airways and obtaining various sizes of blockage samples. As a result, we developed a Google TensorFlow deep learning program that recognizes bronchial blockages through sound recordings at a >75% success rate. Through the experiments, the deep learning program had a 99.28% recognition rate when tested on eight representative blockages, demonstrating the potential of sound-based deep learning programs as a method of bronchial blockage analysis to diagnose respiratory illnesses.
This article has been tagged with: