Recognition of animal body parts via supervised learning
(1) Newton North High School, Newton, Massachusetts, (2) Robert J. and Nancy D. Carney Institute for Brain Science, Brown University, Providence, Rhode Island; Department of Cognitive, Linguistic and Psychological Sciences, Brown University, Providence, Rhode Island
https://doi.org/10.59720/23-109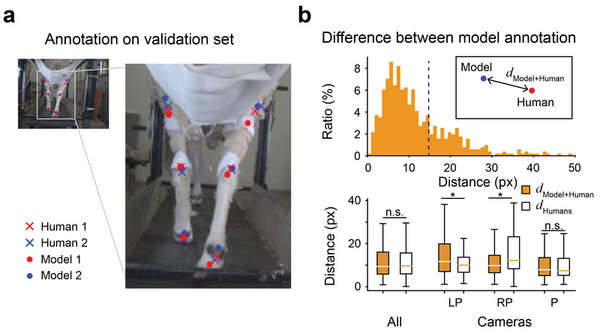
The application of machine learning techniques has facilitated the automatic annotation of behavior in video sequences, offering a promising approach for ethological studies by reducing the manual effort required for annotating each video frame. Nevertheless, before solely relying on machine-generated annotations, it is essential to evaluate the accuracy of these annotations to ensure their reliability and applicability. While it is conventionally accepted that there cannot be a perfect annotation, the degree of error associated with machine-generated annotations should be commensurate with the error between different human annotators. The authors hypothesized that machine learning supervised with adequate human annotations would be able to accurately predict body parts from video sequences. The authors conducted a comparative analysis of the quality of annotations generated by humans and machines for the body parts of sheep during treadmill walking. For human annotation, two annotators manually labeled six body parts of sheep in 300 frames. To generate machine annotations, the authors employed the state-of-the-art pose-estimating library, DeepLabCut, which was trained using the frames annotated by human annotators. As expected, the human annotations demonstrated high consistency between annotators. Notably, the machine learning algorithm also generated accurate predictions, with errors comparable to those between humans. They also observed that abnormal annotations with a high error could be revised by introducing Kalman Filtering, which interpolates the trajectory of body parts over the time series, enhancing robustness. Their results suggest that conventional transfer learning methods can generate behavior annotations as accurate as those made by humans, presenting great potential for further research.
This article has been tagged with: