Comparison of the ease of use and accuracy of two machine learning algorithms – forestry case study
(1) Nord Anglia International School, Dubai, United Arab Emirates, (2) Dubai College, Dubai, United Arab Emirates, (3) NTT Data Services, Chennai
https://doi.org/10.59720/20-156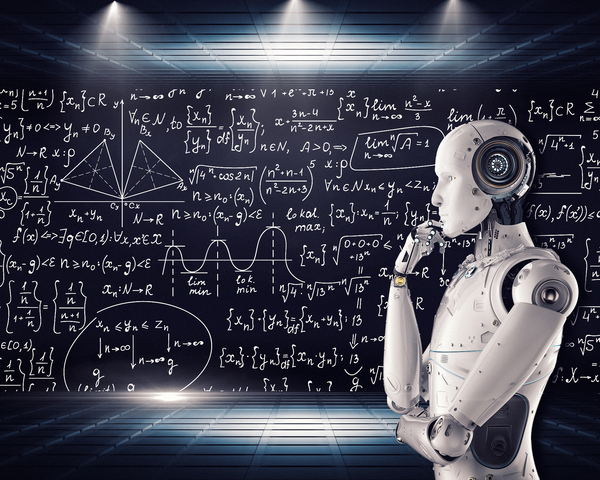
With the availability of massive amounts of data and cheap computing, machine learning has become increasingly viable to create extensive multivariate mathematical models of natural phenomena to help predict accurate future trends that would have been impossible for humans to accomplish by themselves. There is a wide variety of different machine learning algorithms available, and it is not always known which one will perform best for a given dataset. This can be determined after training and evaluating the different models and comparing them. In this case study, logistic regression and random forest models were compared in terms of accuracy and ease of use. We hypothesized that logistic regression would yield a higher accuracy and be easier to set up in a comparable scenario for a given dataset compared to random forest. Both algorithms used the same forestry dataset to see which one would outperform the other. Initially, logistic regression looked like the better choice, however, after a variety of comparisons, random forest yielded higher performance in both accuracy measurements (accuracy=0.9722, Fbeta=0.9722 for random forest vs. accuracy=0.7141, Fbeta=0.6990 for logistic regression) and did not require as much detailed tuning as logistic regression did.
This article has been tagged with: